
In the world of predictive analytics, few techniques have seen such rapid growth and adoption as Support Vector Machines (SVMs). The advent of machine learning and artificial intelligence (AI) has reshaped how businesses and industries harness data to predict outcomes. Among the variety of machine learning algorithms, SVMs stand out due to their robust performance in classification and regression tasks. With 2024 unfolding, SVMs are increasingly revolutionizing predictive analytics, offering more precise, reliable, and actionable insights than ever before. This article dives deep into the rise of SVMs and how they are transforming the landscape of predictive analytics.
What Are Support Vector Machines?
Support Vector Machines are a type of supervised machine learning algorithm that excels in both classification and regression challenges. Developed by Vladimir Vapnik and his colleagues in the 1990s, SVMs are fundamentally different from many other machine learning models due to their ability to find an optimal boundary between data points of different classes. This optimal boundary, known as a hyperplane, is the crux of the SVM’s power, allowing it to classify data points with minimal error.
The algorithm works by mapping data into a high-dimensional space and finding the maximum margin hyperplane that separates the classes. In cases where a clear separation is not possible, SVMs use a kernel trick to transform the data into a higher-dimensional space, making the separation easier.
Why SVMs Outshine Other Algorithms in Predictive Analytics
While there are many machine learning algorithms available—such as Random Forests, Decision Trees, and Neural Networks—SVMs offer several distinct advantages that make them particularly suited for predictive analytics:
- Effective in High-Dimensional Spaces: SVMs perform well in scenarios where the number of dimensions (features) exceeds the number of samples. This is particularly useful in fields like bioinformatics and text classification.
- Memory Efficiency: Unlike other models that require storing all data points, SVMs only rely on a subset of the training data, known as support vectors, which reduces memory usage and improves efficiency.
- Robustness Against Overfitting: By focusing on maximizing the margin between classes, SVMs are less prone to overfitting, particularly in low-noise datasets.
- Versatility with Kernels: The kernel trick enables SVMs to handle non-linear data by transforming it into a higher-dimensional space, making them versatile in tackling complex prediction problems.
The Role of SVMs in Predictive Analytics in 2024
In 2024, the demand for predictive analytics has surged as organizations strive to make data-driven decisions in real-time. From healthcare to finance, predictive analytics is helping businesses anticipate future events and trends, allowing them to optimize operations, reduce costs, and enhance customer experiences. Here’s how Support Vector Machines are playing a pivotal role in this transformation:
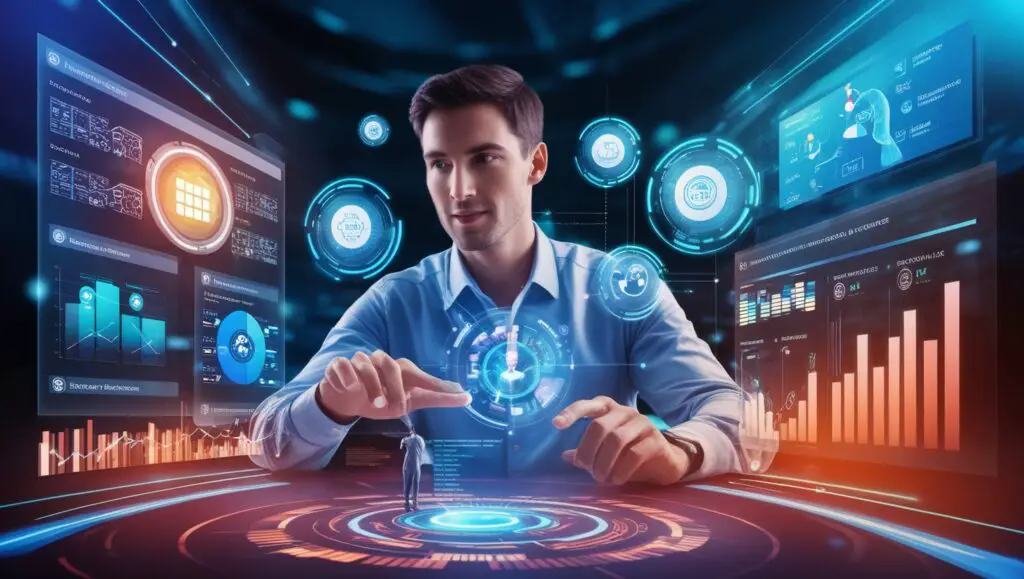
1. Healthcare: Enhancing Predictive Diagnostics
One of the most impactful applications of SVMs in predictive analytics is in healthcare. With the rise of precision medicine, predictive diagnostics have become crucial in identifying potential health risks before they manifest into serious conditions. SVMs are now used to analyze vast amounts of patient data—ranging from genomic data to medical imaging—to predict the likelihood of diseases like cancer, diabetes, and cardiovascular conditions. The accuracy and efficiency of SVMs make them an invaluable tool for early diagnosis and personalized treatment plans.
2. Finance: Revolutionizing Risk Management
In the finance sector, predictive analytics plays a crucial role in managing risks, detecting fraud, and optimizing investment strategies. SVMs are used extensively to analyze historical financial data and predict market trends, credit risks, and customer behavior. For instance, SVMs can be used to predict stock price movements by analyzing patterns in historical price data, which helps investors make informed decisions. Moreover, SVMs have proven effective in detecting fraudulent activities by identifying anomalies in transaction data, thereby safeguarding financial institutions from potential threats.
3. Retail: Optimizing Demand Forecasting
Retail businesses are leveraging predictive analytics to forecast customer demand, optimize inventory, and personalize marketing efforts. SVMs are helping retailers predict which products will be in demand, when customers are likely to make purchases, and how much inventory should be stocked. By accurately predicting customer behavior, retailers can reduce excess inventory, minimize stockouts, and enhance overall operational efficiency. Profitability and customer satisfaction both rise as a result of this.
4. Marketing: Personalizing Customer Engagement
In the world of digital marketing, personalization is key to driving customer engagement and conversions. SVMs are being used to analyze vast amounts of customer data—from browsing behavior to purchase history—to predict what products or services a customer is likely to be interested in. By segmenting customers based on their preferences and behaviors, businesses can tailor their marketing efforts to individual customers, resulting in more personalized and effective campaigns. This level of precision in targeting helps businesses achieve higher conversion rates and stronger customer loyalty.
5. Manufacturing: Predictive Maintenance
In the manufacturing industry, predictive maintenance is a critical application of predictive analytics that helps reduce downtime and improve operational efficiency. SVMs are being used to predict when machinery or equipment is likely to fail by analyzing sensor data and historical maintenance records. By identifying potential issues before they occur, manufacturers can schedule maintenance activities proactively, reducing the risk of unexpected breakdowns and costly repairs.
How SVMs Are Evolving in 2024
The evolution of Support Vector Machines in 2024 is marked by several key advancements that are further enhancing their capabilities in predictive analytics. These advancements are driven by the increasing complexity of data, the demand for real-time predictions, and the integration of AI and deep learning techniques.
1. Integration with Deep Learning
One of the most significant trends in 2024 is the integration of Support Vector Machines with deep learning models. By combining the strengths of both approaches, businesses can leverage the high-dimensional feature extraction capabilities of deep learning with the robust classification performance of SVMs. This integration is particularly useful in complex tasks like image recognition, natural language processing, and speech analysis, where deep learning excels in feature extraction, and SVMs provide precise classification.
2. Enhanced Scalability
As businesses handle larger datasets, the need for scalable machine learning algorithms has become paramount. SVMs are evolving to meet this demand by incorporating distributed computing and parallel processing techniques. These advancements enable SVMs to handle massive datasets efficiently, making them more accessible to industries dealing with big data.
3. Real-Time Predictive Analytics
With the increasing demand for real-time insights, SVMs are being optimized for real-time predictive analytics. Businesses are now able to deploy SVM models in real-time environments, where they can process data as it arrives and generate immediate predictions. This capability is particularly valuable in industries like finance, healthcare, and e-commerce, where timely decision-making is critical.
The Future of Support Vector Machines in Predictive Analytics
Looking ahead, the future of Support Vector Machines in predictive analytics appears promising. As businesses continue to adopt data-driven strategies, the need for accurate and reliable predictive models will only grow. SVMs, with their proven track record of performance and adaptability, are well-positioned to remain a cornerstone of predictive analytics in the years to come.
The continued integration of AI, deep learning, and big data will likely lead to further advancements in SVM technology, enabling businesses to tackle even more complex and high-dimensional prediction problems. Additionally, as industries continue to embrace automation, the demand for predictive maintenance, risk management, and personalized customer experiences will drive the adoption of SVMs across a wide range of applications.
Conclusion
Support Vector Machines are undoubtedly revolutionizing the field of predictive analytics in 2024. From healthcare to finance, retail, marketing, and manufacturing, SVMs are enabling businesses to make more informed decisions, optimize operations, and enhance customer experiences. As SVM technology continues to evolve, its impact on predictive analytics will only deepen, helping businesses unlock new opportunities and stay ahead of the competition.
Thanks for reading!
If you enjoyed this article and would like to receive notifications for my future posts, consider subscribing . By subscribing, you’ll stay updated on the latest insights, tutorials, and tips in the world of data science.
Additionally, I would love to hear your thoughts and suggestions. Please leave a comment with your feedback or any topics you’d like me to cover in upcoming blogs. Your engagement means a lot to me, and I look forward to sharing more valuable content with you.
Subscribe and Follow for More